作為實現人工智慧 (AI) 中重要技術的機器學習 (ML) 在近年展現出強大的技術突破與使用性,許多科學研究開始導入ML來協助,例如透過自動化提高資料獲取的數量或是顯示卡 (GPU) 的加速運算提升效能等。ML在地質碳封存 (GCS) 領域裡也逐漸受到重視,開始有研究透過ML 的幫助來取得成果。
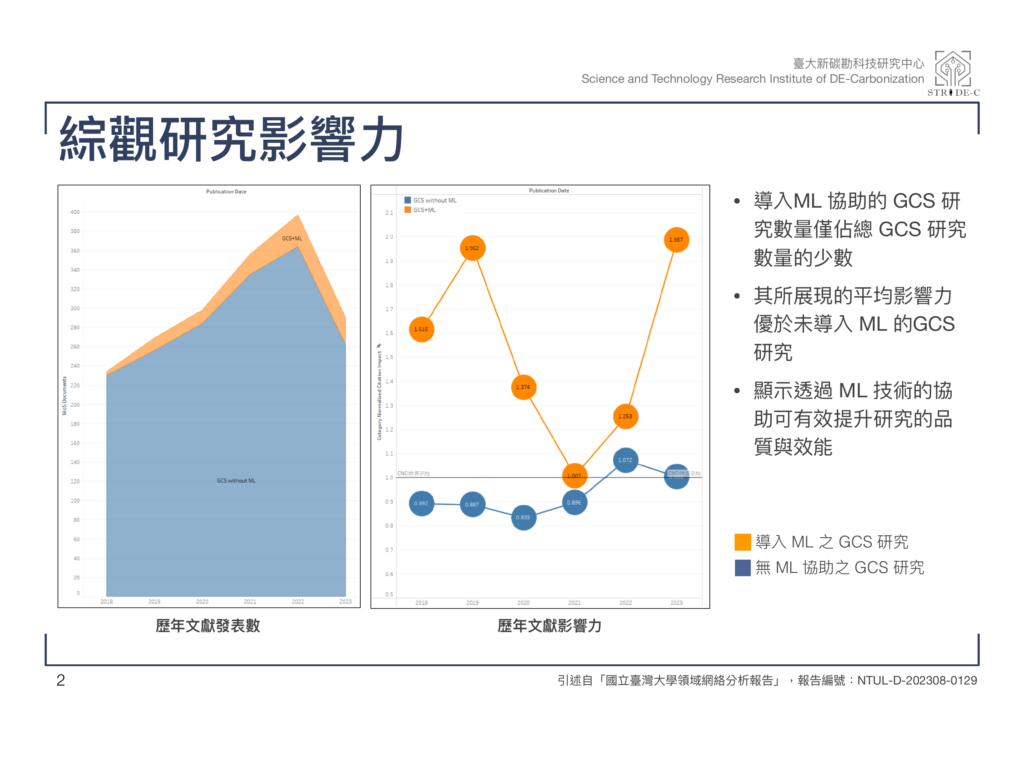
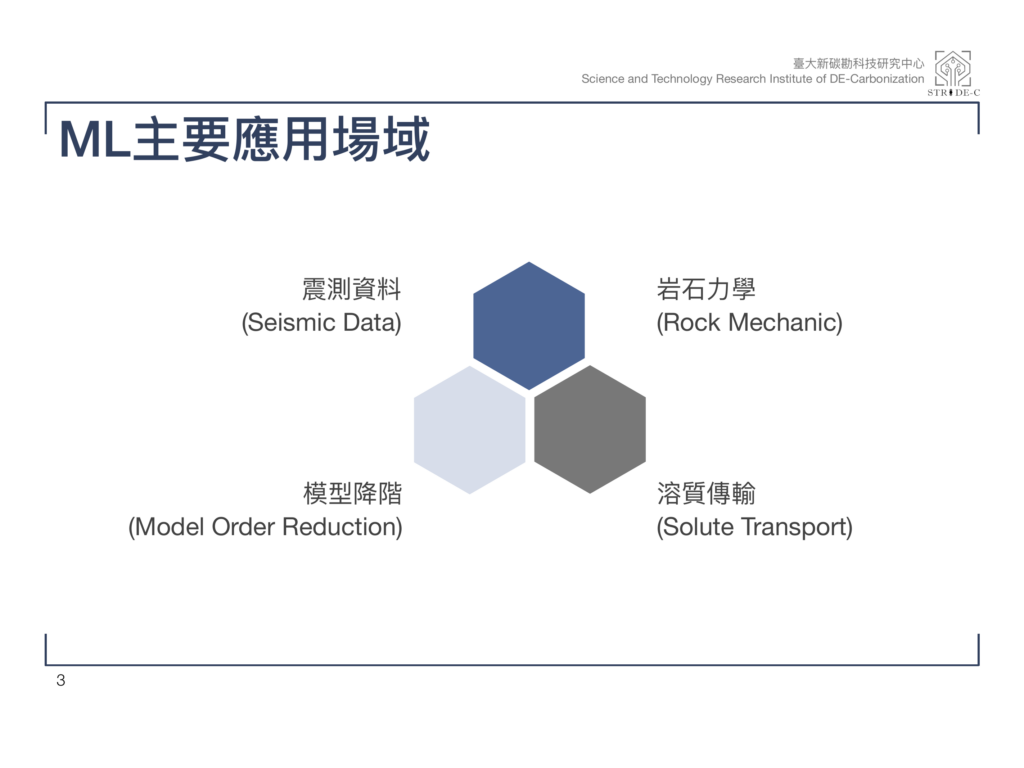
台大領域網絡分析報告指出,於2018-2023年間,導入ML 協助的 GCS 研究數量尚僅佔總 GCS 研究數量的少數;然其所展現的平均文獻影響力 (CNCI) 優於未導入 ML 的GCS研究,顯示透過 ML 技術的協助是可以有效提升研究的品質與效能。
這些ML的應用多集中於模型降階 (Model Order Reduction)、溶質傳輸 (Solute Transport)、震測資料 (Seismic Data)、岩石力學 (Rock Mechanic) 等範疇。本中心正著力於開發震波的自動辨識,目標從密集地震網中辨識出微小地震並快速處理龐大的地震資料,如此便可以提供高解析度的地下構造模型來作為前期地質探勘與中後期工程監測使用。另外亦開發岩相自動辨識的模型,能夠使用岩心圖片或地化資料來快速數位化岩心特性,並標記關鍵岩心區段供專家做進一步的分析辨識。近期也與史丹佛大學團隊合作嘗試導入其傅立葉神經運算子技術,建立深度學習模型來提昇CO2灌注後移棲等特性模擬的運算效能。
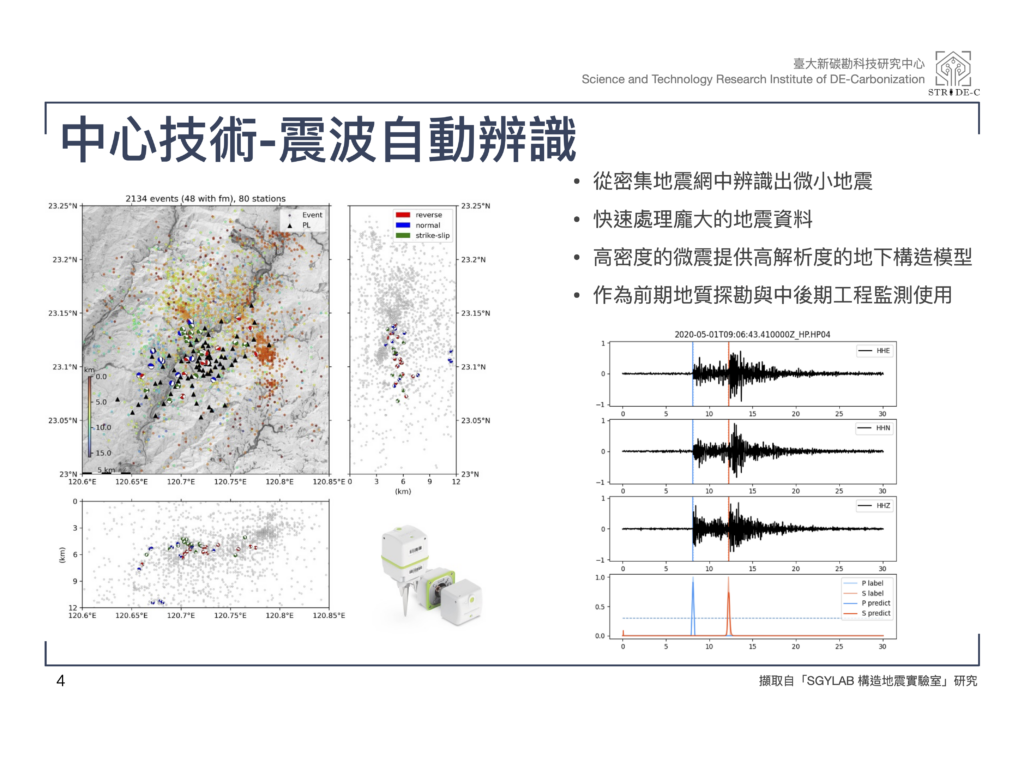
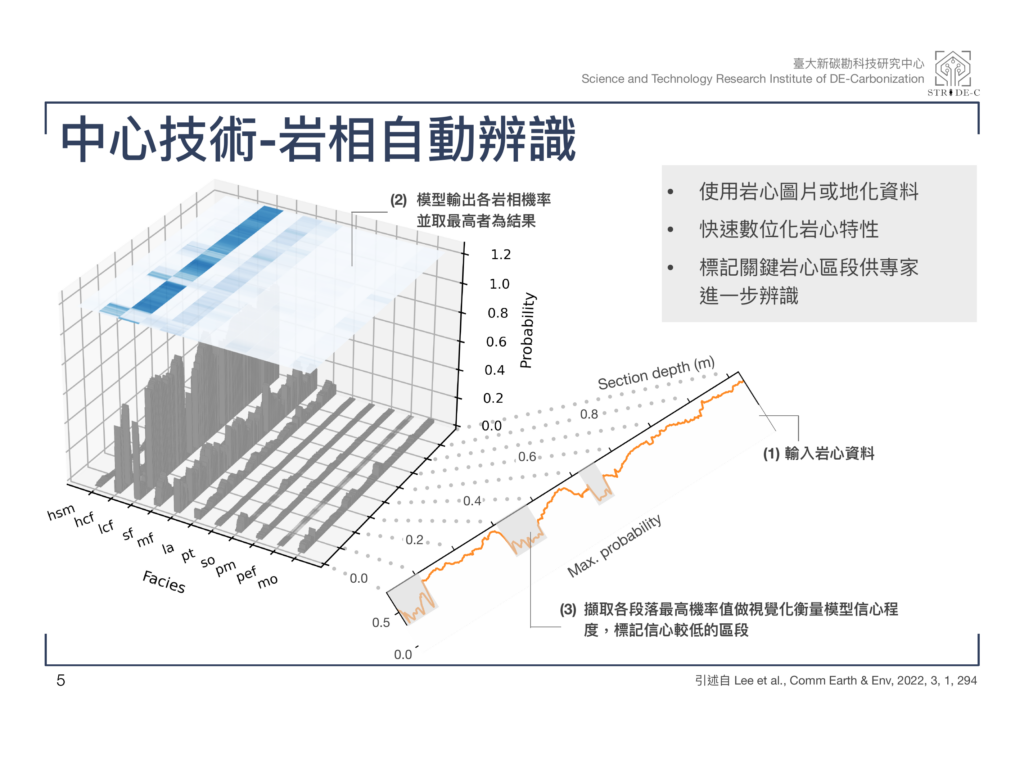
期許未來能夠利用ML技術提供更高效與精細度的碳封存研究。

資料來源:
- 國立臺灣大學圖書館研究支援組(2023)。國立臺灣大學領域網絡分析報告: [ Machine Learning in GCS ] 。國立臺灣大學圖書館。
- Lee, A.-S., et al. (2022). “An automatic sediment-facies classification approach using machine learning and feature engineering.” Communications Earth & Environment 3(1): 294.
- Wen, G., et al. (2023). “Real-time high-resolution CO2 geological storage prediction using nested Fourier neural operators.” Energy & Environmental Science 16(4): 1732-1741.